Getting your Trinity Audio player ready...
|
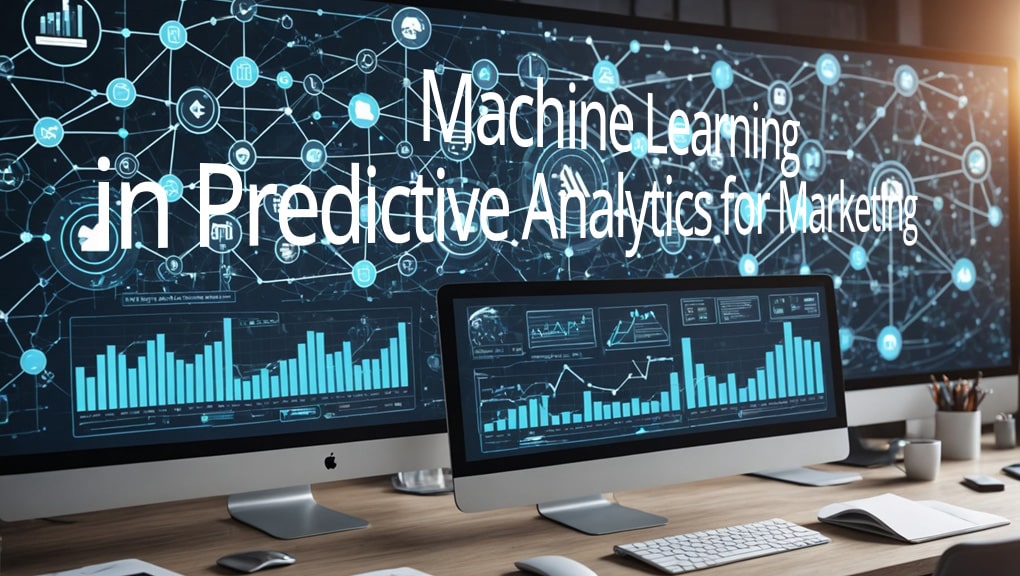
Amid the ever-increasing influx of data and shifting consumer preferences, businesses are constantly on the lookout for innovative ways to stay ahead. Predictive analytics, powered by machine learning (ML), is at the forefront of this revolution, offering a transformative approach to understanding and anticipating consumer behavior. By leveraging the vast amounts of data generated daily, companies can optimize their marketing strategies, personalize customer experiences, and achieve a significant competitive advantage.
Understanding Predictive Analytics in Marketing
Predictive analytics involves using historical data, statistical algorithms, and machine learning techniques to identify the likelihood of future outcomes based on past behavior. In the marketing context, it allows businesses to predict consumer behavior, forecast sales, identify market trends, and make data-driven decisions.
The Role of Machine Learning in Predictive Analytics
Machine learning, a subset of artificial intelligence (AI), enables systems to learn from data, identify patterns, and make decisions with minimal human intervention. When applied to predictive analytics, ML models can process and analyze vast datasets to uncover insights that traditional methods might miss. This capability is particularly valuable in marketing, where understanding consumer preferences and behaviors can significantly enhance campaign effectiveness.
Key Applications of Machine Learning in Predictive Analytics for Marketing
- Customer Segmentation
One of the foundational aspects of effective marketing is understanding different customer segments. Machine learning algorithms can analyze demographic, behavioral, and transactional data to group customers with similar characteristics. These segments allow marketers to tailor their strategies to specific groups, enhancing the relevance and impact of their campaigns.
For example, clustering algorithms like K-means can segment customers based on purchasing behavior, while decision trees can identify which characteristics are most predictive of a customer’s likelihood to respond to a particular offer. - Churn Prediction
Retaining existing customers is often more cost-effective than acquiring new ones. Machine learning models can predict which customers are likely to churn (i.e., stop using a product or service) by analyzing patterns in their behavior. Techniques such as logistic regression, random forests, and neural networks can identify at-risk customers based on factors like engagement levels, purchase frequency, and customer service interactions.
Once identified, businesses can take proactive measures to retain these customers through targeted offers, personalized communication, or improved service quality. - Personalized Marketing
Personalization is a key driver of customer engagement and loyalty. Machine learning models can analyze individual customer data to deliver highly personalized marketing messages. Collaborative filtering, content-based filtering, and hybrid recommender systems can suggest products or content tailored to each customer’s preferences and past behavior.
For instance, e-commerce platforms like Amazon use ML algorithms to recommend products based on a customer’s browsing and purchase history, significantly enhancing the shopping experience. - Sales Forecasting
Accurate sales forecasting is crucial for inventory management, budgeting, and strategic planning. Machine learning models can analyze historical sales data, market trends, and external factors (such as economic indicators) to predict future sales. Techniques like time series analysis, regression models, and neural networks are commonly used for this purpose.
By providing more accurate forecasts, businesses can optimize their inventory levels, reduce stockouts or overstock situations, and make informed strategic decisions. - Customer Lifetime Value (CLV) Prediction
Understanding the long-term value of customers helps businesses prioritize their marketing efforts and allocate resources effectively. Machine learning models can predict the CLV of individual customers based on their purchasing behavior, engagement levels, and other relevant factors. Techniques such as regression analysis and survival analysis are often used to estimate CLV.
By identifying high-value customers, businesses can focus on nurturing these relationships through personalized offers, loyalty programs, and enhanced customer service. - Ad Targeting and Optimization
Digital advertising is a significant investment for many businesses, and optimizing ad spend is crucial for maximizing ROI. Machine learning models can analyze data from various sources (such as website analytics, social media, and ad campaigns) to identify the most effective targeting strategies. Algorithms like logistic regression, support vector machines, and neural networks can predict which users are most likely to engage with an ad or convert.
Additionally, ML models can optimize ad creatives, placement, and bidding strategies in real-time, ensuring that ad spend is used efficiently and effectively. - Sentiment Analysis and Social Listening
Understanding customer sentiment is essential for managing brand reputation and improving customer experiences. Machine learning models can analyze text data from social media, reviews, and customer feedback to gauge public sentiment toward a brand or product. Natural language processing (NLP) techniques, such as sentiment analysis and topic modeling, are commonly used for this purpose.
By monitoring social sentiment, businesses can identify emerging issues, respond to customer concerns promptly, and adapt their marketing strategies to align with public perception.
Case Studies and Real-World Examples
- Netflix: Personalization at Scale
Netflix is renowned for its personalized content recommendations, powered by machine learning. The company uses a combination of collaborative filtering and deep learning models to analyze viewing history, user ratings, and behavior data. These insights enable Netflix to suggest content that aligns with each user’s preferences, driving higher engagement and retention.
By continually refining its recommendation algorithms, Netflix ensures that users discover relevant content, enhancing their overall experience and reducing churn. - Amazon: Predictive Product Recommendations
Amazon’s recommendation engine is a cornerstone of its e-commerce success. The company employs a variety of machine learning techniques, including collaborative filtering, to predict which products a customer is likely to purchase based on their browsing and purchase history. These recommendations are displayed on the homepage, product pages, and marketing emails, driving significant increases in sales and customer satisfaction. - Spotify: Enhancing User Experience Through Personalization
Spotify leverages machine learning to personalize the music listening experience for its users. The platform’s recommendation algorithms analyze user behavior, listening history, and music preferences to create personalized playlists like Discover Weekly and Daily Mix. These curated playlists help users discover new music that aligns with their tastes, enhancing engagement and loyalty.
Additionally, Spotify uses predictive analytics to optimize its marketing campaigns, targeting users with personalized offers and promotions based on their listening habits and preferences. - Starbucks: Predicting Customer Preferences
Starbucks utilizes machine learning to predict customer preferences and optimize its marketing strategies. The company’s loyalty program app collects data on customer purchases, location, and preferences. Machine learning models analyze this data to deliver personalized offers and recommendations through the app.
For example, Starbucks can predict which customers are likely to be interested in a new product launch and target them with tailored promotions. This personalized approach helps drive sales and enhance customer loyalty.
Challenges and Considerations in Implementing Machine Learning for Predictive Analytics
While the benefits of machine learning in predictive analytics for marketing are substantial, there are several challenges and considerations that businesses must address to maximize their success.
- Data Quality and Integration
Machine learning models rely on high-quality, comprehensive data to make accurate predictions. Businesses must ensure that their data is clean, consistent, and integrated from various sources. Data silos and poor data quality can hinder the effectiveness of predictive analytics efforts. - Model Selection and Validation
Choosing the right machine learning model for a specific marketing application is crucial. Businesses must experiment with different algorithms, tune hyperparameters, and validate model performance to ensure accuracy and reliability. Cross-validation and A/B testing are common techniques used to evaluate model effectiveness. - Interpretability and Transparency
Machine learning models, especially complex ones like deep learning, can sometimes be challenging to interpret. Businesses must balance model complexity with interpretability to ensure that marketing teams can understand and trust the predictions. Explainable AI techniques, such as SHAP values and LIME, can help enhance model transparency. - Privacy and Ethical Considerations
Collecting and analyzing customer data raises important privacy and ethical considerations. Businesses must comply with data protection regulations (such as GDPR and CCPA) and ensure that they handle customer data responsibly. Transparency about data usage and obtaining customer consent are essential for maintaining trust. - Scalability and Deployment
Implementing machine learning models at scale requires robust infrastructure and efficient deployment strategies. Businesses must invest in scalable cloud-based solutions, automate model training and deployment pipelines, and monitor model performance in real-time to ensure scalability and reliability. - Continuous Learning and Adaptation
Consumer behavior and market dynamics are constantly evolving. Machine learning models must be continuously updated and retrained with new data to maintain their accuracy and relevance. Businesses must establish processes for ongoing model monitoring, retraining, and adaptation to changing market conditions.
Future Trends in Machine Learning for Predictive Analytics in Marketing
As technology continues to evolve, several emerging trends are shaping the future of machine learning in predictive analytics for marketing:
- Deep Learning and Neural Networks
Deep learning techniques, particularly neural networks, are becoming increasingly prevalent in predictive analytics. These models excel at processing large volumes of complex data and can uncover intricate patterns that traditional methods might miss. Deep learning is expected to drive advancements in personalization, recommendation systems, and customer behavior prediction. - Real-Time Predictive Analytics
The demand for real-time insights is growing as businesses seek to respond swiftly to changing customer behavior and market conditions. Real-time predictive analytics powered by machine learning allows companies to make instant data-driven decisions, optimize marketing campaigns in real-time, and deliver timely personalized experiences. - Integration with IoT and Edge Computing
The proliferation of Internet of Things (IoT) devices is generating massive amounts of real-time data. Machine learning models can analyze this data to gain valuable insights into consumer behavior and preferences. Edge computing, which processes data closer to the source, enables faster and more efficient predictive analytics, particularly for IoT applications. - AI-Powered Customer Interaction
The integration of AI-powered chatbots and virtual assistants in marketing is enhancing customer interaction and support. These systems use natural language processing and machine learning to understand customer queries, provide personalized responses, and predict customer needs. AI-driven customer interaction is expected to play a significant role in improving customer satisfaction and driving sales. - Ethical AI and Fairness
As machine learning becomes more pervasive in marketing, there is an increasing focus on ethical AI and fairness. Businesses must ensure that their models do not inadvertently perpetuate biases or discrimination. Techniques for detecting and mitigating bias in machine learning models are gaining importance, promoting fairness and inclusivity in marketing practices.
Final Thoughts
Machine learning in predictive analytics is revolutionizing the marketing landscape by providing businesses with powerful tools to understand and anticipate consumer behavior. From customer segmentation and churn prediction to personalized marketing and sales forecasting, machine learning models offer unprecedented insights that drive smarter marketing strategies and enhance customer experiences.
While the journey to implementing machine learning in predictive analytics comes with challenges, the potential benefits are immense. By leveraging high-quality data, selecting appropriate models, ensuring transparency and ethical practices, and embracing emerging trends, businesses can unlock the full potential of machine learning to stay ahead in the competitive market.
As technology continues to advance, the integration of machine learning in marketing will only deepen, enabling businesses to forge stronger connections with their customers, optimize their marketing efforts, and achieve sustainable growth in an ever-evolving digital world.
P.S. If you’re looking to elevate your digital presence and navigate your own journey to success, reach out to Cosmos Revisits for tailored marketing strategies that can help you achieve your goals.